Current Grants and Projects:
Collaborative Research: MRA: Climate legacies and timescales of influence on carbon cycle processes in drylands
PI = Kiona Ogle; Co-PIs (NAU) = Jarrett Barber, Drew Peltier, Kimberly Samuels-Crow, Debbie Huntzinger; Co-PIs (UCLA) = Nicholas Parazoo, Rong Fu. This study seeks to quantify legacies (prolonged impacts of an extreme event) and memory (timescales of influence of drivers, whether extreme or not) of climate-related drivers on key C cycle components, over a range of spatial and temporal scales across drylands in the western US. This study will (1) quantify the magnitude and duration of climate (e.g., drought) legacies on C fluxes in the western US; (2) evaluate climatic memory and the timescales over which climate drivers impact C fluxes; (3) determine how legacy and memory responses vary across drylands in the western US; and (4) evaluate the importance of legacies and memory for the C cycle. To address these, this study will employ modern statistical methods (wavelet analyses, machine learning, Bayesian models) to integrate diverse datasets varying in temporal and spatial coverage and resolution, including multiple tree-ring networks, ground-based flux towers, satellite data products, model-data fusion products, and biospheric model outputs. This study will provide insights into the magnitude and duration of climate-C cycle legacies, how these legacies vary across space, and the potential mechanisms giving rise to such legacies. The project includes multiple training and outreach activities aimed at the scientific community, land and resource managers, agency stakeholders, and the public.
NRT-HDR: T3: A new Team-based, T-shaped scientist Training paradigm integrating informatics and ecology. Funded by the National Science Foundation (NSF) Graduate Traineeship (NRT) Program.
PI = Kiona Ogle; Co-PIs (all NAU) = Jarrett Barber, Andrew Richardson, Teki Sankey, Ben Ruddell; Senior Personnel (all NAU) = Paul Flikkema, Scott Goetz, Chris Doughty, Bruce Hungate; Evaluator = Pam Bishop (U Tennessee). Project Summary: This NRT training program is couched in the interdisciplinary research theme of understanding and forecasting the properties and processes of terrestrial ecosystems, especially in the context of understanding the impact of and feedbacks to global change. The field is being flooded with data (e.g., from various ecological and Earth observatory networks) that are critical to understanding and forecasting terrestrial ecosystem responses. However, most graduate students and scientists lack the quantitative, computational, and collaborative skills to leverage such data to address grand, challenging questions. This NRT program will train the next generation of scientists to overcome these limitations, with a focus on training in informatics tools and team-science skills that facilitate solutions to complex problems, motivated by the question: How does anthropogenic global change affect the health and productivity of terrestrial ecosystems? In this innovative graduate training program, trainees will create their own T-shaped scientist profile in ecological and environmental informatics (EEI) by building substantive depth (stem of T) in a primary core competency and establishing breadth (bar of T) in other primary and supporting areas. The primary areas include hard skills in data analytics and computing, analysis and synthesis, and terrestrial ecosystem science; the supporting areas include relevant data collection tools and products and soft skills in communication, collaboration, and team building. Elements of this NRT program are scalable and sustainable, including: (1) a curriculum that allows students to create their personalized T-profile, supported by existing and new classes and a variety of training activities, (2) workshops, courses, and other activities that focus on teaming skills and applying these skills to collaborative research projects, (3) preparation for a range of careers via professional development and applied internship opportunities, and (4) the graduate EEI certificate that is accessible to students in a range of STEM fields. These program components are expected to benefit MS and PhD students in multiple academic units at NAU, and the program will serve as a role model that can be transferrable to other emerging informatics programs across the US.
Collaborative Research: Ecohydrological controls on evapotranspiration across a semiarid elevation gradient
PI = Kimberly Samuels-Crow (NAU); Co-PIs = Kiona Ogle (NAU), Marcy Litvak (U New Mexico), and John Bradford (USGS). Evapotranspiration (ET) represents a major component of water budgets in semiarid ecosystems, but quantifying controls on ET is hampered by a shortage of long-term measurements, and models have difficulty accurately predicting ET in these systems. We will address this gap by quantifying the relative importance and timescales of influence of environmental drivers of ET and its components (evaporation, E, and transpiration, T) by testing three main hypotheses across six semiarid ecosystems common to the region: (1) evaporation and transpiration dominate ET at different times of the growing season, and provide insights into which processes are important at different times, (2) evaporation and transpiration are sensitive to different combinations of environmental drivers, and the temporal relationships between these components of ET and their environmental drivers vary within and between ecosystems, and (3) past environmental conditions are important for governing ET, especially the transpiration component, and the importance of past conditions is amplified at more arid sites. Partitioning ET into its components (evaporation and transpiration) lies at the heart of the proposed work, but partitioning ET using different methods can lead to conflicting results. Therefore, in each ecosystem, we will take the innovative approach of using two to five methods to partition evaporation and transpiration and then reconcile contradictory results using a Bayesian statistical approach. We will rely on a combination of existing ET and sap flow (a proxy for transpiration) measurements, new measurements of isotopes in water in soil, stems, and the atmosphere, and modeling techniques to partition ET, to identify important drivers, and to evaluate the timescales over which these drivers control ET. These results will be incorporated into an existing process-based model (SOILWAT) to evaluate their implications for ecosystem level water budgets across the sites and to simulate the impact of varying the environmental conditions on local water budgets.
Past Grants and Projects:
Lags and legacies: antecedent effects on grassland biomass response to CO2. Funded by the Australian Research Council.
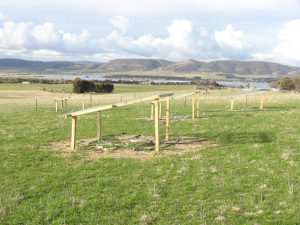
PIs = Mark Hovenden (U Tasmania) and Martin De Kauwe (U of New South Wales). Unfunded collaborator = Kiona Ogle. Summary of project: Grassland accounts for ~41% of Australia’s agricultural production, and variation over time in grassland productivity is largely driven by water availability and associated changes in nutrient supply. Rising atmospheric CO2 is perhaps the most reliable and consistent global change factor likely to affect terrestrial ecosystem productivity. Rising CO2 should stimulate productivity almost universally and this influence is embedded in land-atmosphere and global climate models. However, the average biomass response to elevated CO2 (eCO2) in grassland experiments is much lower than in crops and hugely variable between years. Current production models fail to represent this variation in the effect of eCO2 on grassland productivity because understanding of the specific drivers of these responses is currently lacking. This lack of knowledge impedes progress on modelling land-atmosphere carbon fluxes thereby limiting the accuracy of global climate models. We have an approach – based on how past conditions affect the current response to eCO2 – that will substantially advance our understanding of what controls grassland biomass responses to rising CO2, which will allow us to improve our ability to predict the impacts of future CO2 on grassland productivity. We base this new approach on preliminary data analyses and recent experimental results collected by the PIs from the TasFACE2 experiment, which indicate that both short- (months to 1-2 years) and long-term (years to decades) legacy effects of past climatic conditions could influence the productivity response to CO2. This project aims to determine how past conditions influence current responses to eCO2 by: (1) Using the stochastic antecedent modeling (SAM) approach to uncover how past conditions influence the biomass response to increased CO2 in previous grassland experiments from around the world; (2) Using a grassland FACE experiment (TasFACE2) to determine which facets of the ecosystem are most strongly controlled by past conditions and how these influence the biomass response to eCO2; (3) Applying the SAM approach to unlock the mechanisms that control the strength and extent of antecedent influences on the biomass response to eCO2 in the TasFACE2 experiment.
ABI Innovation: Quantifying, simulating, and visualizing tree growth and its antecedent endogenous and climatic predictors. Funded by NSF.
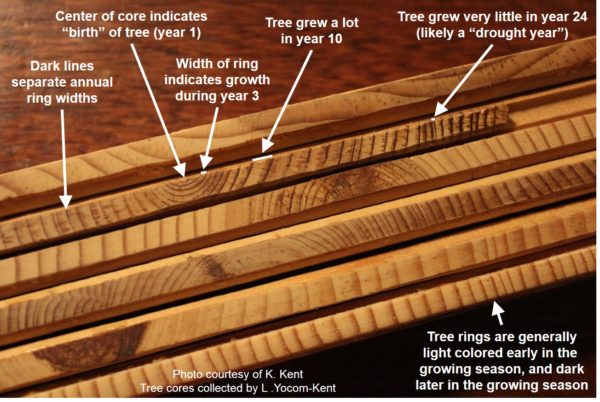
PI = Kiona Ogle; Co-PI = Jarrett Barber (NAU). Summary of project: Environmental conditions averaged over past days, weeks, months, seasons, or years are important predictors of plant and ecosystem productivity. For example, tree-ring studies often show that annual tree growth is correlated with precipitation or temperature during seasonal time periods that align with the timing of ring formation. Although such studies clearly indicate that tree growth is affected by antecedent exogenous (e.g., past climate) and endogenous (e.g., past ring widths) factors, existing analysis methods do explicitly and simultaneously evaluate the role of each factor. This study aims to simultaneously quantify the antecedent endogenous and exogenous (climate) factors governing tree growth. Four objectives study are: (1) Develop a stochastic antecedent model (SAM) for quantifying antecedent climatic and endogenous conditions and their influence on tree growth. (2) Apply the SAM to estimate the time-scales over which antecedent factors affect tree growth for multiple species across multiple sites. (3) Identify potential physiological mechanisms underlying the antecedent effects on tree growth. (4) Develop software for more general applications of SAM and for simulating and visualizing tree growth. To address these objectives, this study combines large datasets, field studies, literature data, Bayesian synthesis, and an individual-based model (IBM) of tree growth and physiology. The SAM will be implemented via Bayesian methods to synthesize historical climate data and >870,000 tree-ring records for 20 tree species across 405 sites in the Southwest. This analysis will be used to inform a field study that explores linkages between the antecedent responses and tree functional traits related to labile carbon storage. These diverse data will inform the IBM, which will be applied to understand the physiological mechanisms underlying the antecedent effects on tree growth over time and across multiple species and sites.
RAPID: Leveraging the 2015-2016 El Nino to evaluate drought legacy effects on tree growth responses to rare wet events. Funded by NSF.
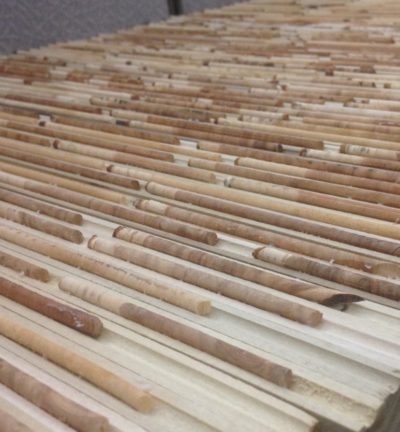
PI = Kiona Ogle; Co-PIs = William Anderegg (Univ Utah), David Auty (NAU), George Koch (NAU), and Marcy Litvak (Univ New Mexico). Summary of project: The current El Niño has produced a rare gradient in winter (Oct 2015-Feb 2016) precipitation across the Four-Corners region, spanning 25% to over 400% of the long-term mean. Four years prior, the 2011-2012 drought significantly and negatively impacted tree populations, soil moisture availability, and ecosystem C sequestration. Recent work has demonstrated that it takes 3-5 years for tree growth, ecosystem C fluxes, and net ecosystem productivity to recover from past, severe droughts. The unprecedented conditions created by this El Niño, including unusually wet conditions, provides a unique and timely opportunity to understand the legacy effects of past droughts on tree growth during rare wet conditions, quantify drought recovery thresholds, and identify the potential physiological mechanisms underlying these responses. The objective of this study is to determine the extent to which past climatic conditions, especially droughts, govern tree physiological and growth responses during wet periods. This study hypothesizes that: 1) Tree responses to wet episodes are governed by past drought severity and tree-level impacts; 2) Legacy effects of drought persist for 3-5 years, thus 2016 growth responses will be independent of the 2000-03 drought, but will be modulated by 2011-12 drought severity; 3) Trees that rely primarily on shallow soil water (generally, summer rains) will show a dampened growth response to current El Niño precipitation compared to those that rely on deeper moisture (likely recharged during winter); 4) Trees more negatively affected by past drought will show reduced physiological function during 2016, leading to reduced ability to respond to current El Niño conditions; 5) Recovery from the 2011-12 drought will exhibit a non-linear, threshold response such that above average precipitation is required to recover to “average” growth.
Collaborative Research: Extreme events and ecological acclimation: Scaling from cells to ecosystems. Funded by NSF.
PI = Steve Pacala (Princeton); Co-PIs = Bill Anderegg (Princeton), Adam Wolf (Princeton), Kiona Ogle (Northern Arizona Univ), Franco Biondi (Univ Nevada, Reno), Chris Schwalm (Northern Arizona Univ), George Koch (Northern Arizona Univ). Summary of project: This project focuses on the legacies of droughts on individuals and communities that comprise ecosystems. Leveraging a unique set of instrumentation, the project is monitoring the continuous physiological variation and recovery of individuals of different dominant tree species in the Southwest in response to drought. This detailed physiology will be linked to an adjunct inventory of the national Forest Inventory and Analysis (FIA) program to understand how physiological responses, which may differ among species, shape subsequent growth and mortality following drought across the broader region. The project is testing whether individual tree responses can be scaled to match fluxes at the ecosystem level indicated by eddy covariance measurements, examining key hypotheses of how these physiological responses scale to a community and ecosystem level in order to improve predictive models of future climatic impacts. Using these data and analyses, this study aims is addressing the question: Does a drought leave an ecosystem weaker or stronger in the face of subsequent drought? The project will directly investigate the recovery timescales and limits across dominant species with divergent life-history strategies and assess the opportunities and barriers to scaling individual physiology to ecosystem measurements.
Building capacity in Bayesian analysis for practicing ecologists. Funded by NSF.
PI = Tom Hobbs (Colorado State Univ); Co-PIs = Kiona Ogle (Northern Arizona Univ), James Clark (Duke Univ), Mevin Hooten (Colorado State Univ), Maria Uriarte (Columbia Univ). Summary of project: Challenging models with observations forms the fundamental route to insight in ecology, but it has become clear that many problems in ecology—problems that cut across all its sub-disciplines—cannot be solved using classical statistical methods. These research problems share a set of four interrelated challenges. First, uncertainty arises in many ways when we try to model ecological systems–our models are too simple; our observations are made imperfectly; there are inherent differences among individuals and locations–and these several sources cannot be combined in a coherent way via classical statistical models. Many problems in ecological research are also informed by multiple sources of data that cannot be aggregated in traditional structures for inference, and we often need to estimate quantities that are inherently unobservable or that can only be observed indirectly. Finally, there is an urgent need to use models to make forecasts about the behavior of the environment, forecasts that include an honest appraisal of uncertainty. Failing to rise to these challenges impedes the development of ecological theory and hinders its useful application to pressing environmental problems. Hierarchical Bayesian methods provide a way to overcome these impediments, allowing investigators to address questions that heretofore were seen as too complex to answer. Many ecologists recognize the promise of these methods but lack an efficient way to learn them. This project will offer intensive training on hierarchical Bayesian methods to post-doctoral researchers, academic faculty, and scientists at agencies and non-governmental organizations. To deliver this training, we are developing and teaching annual, two-week workshops over at least three years. The goals of the training include: (1) Provide a principles-based understanding of Bayesian methods needed to train students, to evaluate papers and proposals, and to solve research problems; (2) Communicate the statistical concepts and vocabulary needed to foster collaboration between ecologists and statisticians, and (3) Provide the conceptual foundations and quantitative confidence needed for self-teaching modern analytical methods.
Data-model synthesis of grassland carbon metabolism: Quantifying direct, indirect and interactive effects of warming and elevated CO2. Funded by the Department of Energy (DOE).
PI = Elise Pendall (Univ of Wyoming); Co-PIs = Kiona Ogle (Northern Arizona Univ), David Williams (Univ Wyoming), Jana Heisler-White (Univ Wyoming), Jack Morgan (USDA-ARS, Ft Collins), Feike Dijkstra (USDA-ARS), William Parton (Colorado State Univ). Summary from proposal: Our project will assess direct, indirect and interactive effects of elevated CO2 and warming on C metabolism and its components at the Prairie Heating and CO2 Enrichment (PHACE) experiment, with particular emphasis on quantifying the role of soil water availability. To achieve this, we will integrate extensive and diverse field observations from a state-of-the art experiment with process models. At the PHACE experiment, we investigate how multiple global change factors interact to influence a native mixed-grass prairie ecosystem by deploying well-replicated, state-of-the-art Free-Air CO2 Enrichment (FACE) and infra-red warming manipulations. Additional shallow (summer) and deep (spring) irrigation treatments allow evaluation of how seasonality of precipitation influences mixed-grass prairie, and how the magnitude of indirect water effects compares to direct effects of CO2 and warming. To understand mechanisms underlying ecosystem responses, we make extensive use of gas exchange, stable isotope, soil water and nitrogen monitoring, and computer simulation models. Results from the first four years (2006-2009) of manipulations have enhanced mechanistic understanding of ecosystem responses to global changes. This project is integrating our detailed field experiments and process-based modeling, which is expected to yield a predictive framework for 1) evaluating how changes in water availability control ecosystem responses to other global changes such as elevated CO2 and temperature and 2) quantifying ecosystem responses that cannot be explained by water availability.
RCN: Forecasts Of Resource and Environmental Changes: data Assimilation Science and Technology (FORECAST). Funded by NSF Research Coordination Network program.
PI = Yiqi Luo (Univ Oklahoma), Co-PIs = James Clark (Duke Univ), Shannon LaDeau (Cary Institute of Ecosystem Studies), Kiona Ogle (Northern Arizona Univ), and David Schimel (NEON Inc.). Note: this grant does not support basic research. Summary from proposal: Several forces are driving a transformation in ecological research towards a science of ecological forecasting. Such forces include: (i) dramatically increased availability of data from observational and experimental networks, (ii) leaps in computational power, (iii) advances in statistical and optimization methodologies, and (iv) the societal need to anticipate global change impacts on our natural resources, and thus develop better strategies for managing these resources. Enhanced and accessible data assimilation approaches are key to improving the accuracy of ecological forecasts. To facilitate this transformation, this proposal describes a network (FORECAST) of activities with the primary objectives of further developing, applying, and promoting ecological forecasting and data assimilation techniques. The FORECAST network will organize conferences related to: (i) data assimilation towards ecological forecasting, (ii) data processing, mining, and assimilation, (iii) uncertainty analysis and information content of data, models, and forecasts, (iv) education, curriculum development, and organization of training programs, and (v) interfacing data assimilation and ecological forecasting with data generated from sensor networks via data archival/retrieval advances in cyberinfrastructure. The FORECAST network has the potential to develop a community of scientists with expertise in data assimilation and ecological forecasting. The ability to forecast changes in biodiversity, infectious disease, and other ecological resources and properties will directly benefit society in terms of preparedness for potential ecological disasters and improvement of resource management under a changing climate.
A theoretical and computational framework for linking tree form and function to forest diversity and productivity. Funded by NSF Advances in Biological Informatics (ABI).
PI = Kiona Ogle; Co-PI = Jarrett Barber (Arizona State Univ). Summary from proposal: Forest ecosystems cover nearly 50% of the Earth’s land area and contain about 90% of the global vegetation carbon. Thus, climate change impacts on forests will ultimately affect biodiversity, productivity, and carbon cycling of the terrestrial biosphere. This study aims to develop and test a scaling framework for understanding forest diversity and productivity, and may be seen as a necessary step in our continuing effort to develop and apply this framework to forecast impacts of climate change on forests. Our proposed work addresses three questions that are paramount to developing this framework and to constructing general theories of how the form and function of individual organisms (e.g., trees) affect large-scale ecological phenomena (e.g., forest dynamics). (1) How variable are functional traits? How do traits related to tree form (e.g., allometries, morphology) and function (e.g., physiology, growth, allocation, survival) vary between species, and how do evolutionary versus environmental drivers affect trait variability? (2) Do species matter? Is a species-specific representation of form and function necessary to accurately describe community and ecosystem properties (e.g., diversity, succession, productivity, carbon cycling)? (3) How do biological processes scale? How do we develop a general scaling framework for predicting large-scale forest dynamics that acknowledges species-specific trait variability, includes key physiological mechanisms, and satisfies mass balance rules? Our approach integrates large and disparate data sources (i.e., literature, forest inventory, and tree-ring width databases), phylogenetic information, and process models of tree growth. Specifically, we propose to develop: (i) process models that link tree form and function, incorporate key plant functional traits, and are applicable to broad spatial and temporal scales; (ii) new meta-analysis methods for analyzing literature information on species-specific traits that incorporate phylogenetic relationships and overcome limitations common to other “standard” approaches (e.g., missing sample sizes, multiple sources of uncertainty, and incomplete covariate data); and (iii) rigorous statistical and computational methods for integrating the large and disparate data sources with the process model. This highly integrative approach will provide a major step towards building and testing a general scaling framework.
Quantifying the importance of aboveground controls on soil carbon efflux in deserts of the Southwest. Funded by DOE National Institute for Climatic Change Research (NICCR)
PI = Kiona Ogle; Collaborators = David Tissue (University of Western Sydney), Travis Huxman (University of Arizona), Stan Smith (University of Nevada, Las Vegas), and Michael Loik (University of California, Santa Cruz). Summary from proposal: This study is part of our ongoing synthesis of data from Southwest deserts to quantify the importance of aboveground processes (e.g., plant carbon assimilation, allocation) to soil respiration (CO2 efflux). We are conducting a rigorous synthesis of existing data and developming and testing process models that incorporate aboveground controls on soil respiration. Previous work has employed phenomenological models that assume soil respiration is affected by soil water and temperature. A fairly novel aspect is the incorporation of previous (antecedent) moisture conditions, which are critical to predicting soil respiration in deserts and appear to reflect aboveground plant activity that differs across deserts (e.g., rooting distributions, timing of activity, species composition). But, the models do not explicitly include aboveground processes and only explain 35-70% of the variation in observed respiration. This study aimed to improve our ability to predict soil carbon fluxes by incorporating aboveground controls. Our current study focuses on five sites representative of the major US deserts (Great Basin, Mojave, Sonoran, Chihuahuan). Studies conducted at each site manipulated water inputs and provided data on carbon and water dynamics of soils, plants, and whole ecosystems, accompanied by soil physicochemical and micro-meteorological data. There is significant overlap in the types of data collected, facilitating cross-site synthesis via hierarchical Bayesian (HB) tools. We compiled all data into a database (DUDES), and we are significantly extending our HB approach to synthesize data in DUDES in the context of process models that include aboveground controls on soil respiration. The respiration models, which range from phenomenological to physical-based diffusion models, will be coupled to biochemical models of leaf gas exchange, semi-mechanistic models of carbon allocation, and physical-based models of soil water. The HB methods offer a statistically rigorous data-model integration framework for answering our primary science questions and inferring the potential impacts of altered precipitation on soil respiration.
Biotic processes regulating the carbon balance of desert ecosystems. Funded by DOE.
PI = Bob Nowak (Univ Nevada, Reno), Co-PIs = Dave Evans (Washington State Univ), Kiona Ogle (Arizona State Univ), Stan Smith (Univ Nevada, Las Vegas), Lynn Fenstermaker (Desert Research Institute). Summary from proposal: This project will integrate and synthesize 10 years of aboveground and belowground (roots and soil) ecosystem process data with the final harvest and carbon stock data collected during the decommissioning of the Nevada Desert FACE Facility (NDFF). Our overall objectives are to develop and apply modern statistical methods for synthesizing existing ecosystem process data from 10 years of the FACE experiment with new data from the final harvest within the context of process-based models developed by Kiona Ogle, University of Wyoming, who would join our team. This data-model synthesis approach will allow us to infer the impacts of elevated CO2, in combination with natural precipitation variability, on multiple, interconnected ecosystem processes related to carbon, water, and nutrient cycling across a range of time scales. Given the unique nature of the NDFF in a desert ecosystem coupled with the contrasting results observed to date at the NDFF, a timely and detailed synthesis of our datasets will provide important insights into carbon cycling and sequestration for desert ecosystems throughout the southwestern US and around the world.
NICCR Focus 4: Synthesis of existing datasets to explore the implications of altered precipitation for carbon and water dynamics in desert ecosystems of the southwestern US. Funded by DOE-NICCR.
(2006-2010) PI = Kiona Ogle; Co-PIs = David Tissue (Univ Western Sydney), Travis Huxman (Univ of Arizona), Stan Smith (Univ of Nevada, Las Vegas), and Michael Loik (Univ of California, Santa Cruz). Summary from proposal: This study is motivated by the potential for altered precipitation regimes (an element of climate change) to greatly impact water-limited ecosystems of the Southwest. Existing field studies exploring the effects of variation in precipitation on C (carbon) and H2O (water) dynamics in deserts of the Southwest have produced large datasets. However, no syntheses have been conducted with respect to these datasets to explore the broader implications of altered precipitation for arid and semiarid ecosystems. Our objective is to synthesize existing data related to C and H2O fluxes, spanning leaves to ecosystems, across four major deserts in the Southwest. A second objective is to use the synthesis results to identify novel questions/hypotheses and to help direct future, cross-site experiments. The datasets are derived from five extensively-studied sites: Santa Rita Experimental Range and San Pedro River Basin, AZ (Sonoran Desert), Big Bend NP, TX (Chihuahuan Desert), Nevada Test Site, NV (Mojave Desert), and Valentine Eastern Sierra Reserve, CA (Great Basin Desert). The Big Bend site occurs in the NICCR Southeastern Region, and the others are in the Western Region. The synthesis results will be used to address a series of questions, including: How are C and H2O cycles affected by changes in pulse, seasonal, and annual precipitation? What ecological components appear critical to ecosystem C and H2O exchange, and which are most sensitive to precipitation changes? With respect to C and H2O, how do the four deserts differ in their responses to altered precipitation? What environmental variables (e.g., nitrogen, temperature) interact with precipitation to strongly affect C and H2O dynamics in these systems? We will synthesis data from these sites within a Bayesian hierarchical framework that facilitates simultaneous coupling of diverse data sources and mechanistic models; the models contain ecologically-meaningful parameters that, when parameterized with field data, provide important insights into the factors controlling C and H2O dynamics at different scales. Feedback to field studies is central to designing experiments that explore effects of multiple global change factors on deserts of the Southwest, and terrestrial ecosystems in general. Products from the study will contribute to building a better understanding of how arid lands may be affected by environmental changes such as altered precipitation.
Unlocking the mysteries of belowground plant form and function. Funded by U of Wyoming Faculty Grant-in-aid.
PI = Kiona Ogle (2010) Summary from proposal: The carbon and water cycles are tightly coupled in arid and semiarid ecosystems, and the belowground system (i.e., plant roots and soil microorganisms) is critical to understanding and predicting ecosystem carbon and water dynamics in these ecosystems. However, the role of the belowground system is less studied and less understood compared to the aboveground system. Hence, the objective of this study is to develop a general theory of the importance of belowground plant form and function (related to root activity) to carbon and water dynamics in semiarid ecosystems. Towards addressing this objective, we are conducting a field experiment in the sagebrush steppe in southeastern Wyoming that uses stable isotope tracers to explore root activity patterns throughout the growing season. Data obtained from the experiment will be analyzed within the context of ecological models that quantify root-soil carbon and water interactions.
Understanding forest responses to climate change: Developing a mechanistic framework of tree and forest carbon dynamics. Funded by Wyoming NASA Space Grant Consortium.
PI = Kiona Ogle; Collaborator = Jarrett Barber (Univ Wyoming, Statistics) (2008-2009). Summary from proposal: This proposal stems from an ongoing collaboration to understand and predict forest dynamics and how these dynamics are affected by climate change. This goal aligns with NASA’s Science of the Earth (SOE) Carbon Cycle and Ecosystems Focus Area goals: quantify global productivity, biomass, and carbon fluxes; document, understand, and project changes in the carbon cycle and terrestrial ecosystems. Moreover, our data-model integration approach aligns with SOE’s strategic principle: develop Earth system models that integrate observations with process modeling to simulate linkages between important processes. Our work focuses on forests because they account for 90% of the carbon stored in vegetation and critical components of the terrestrial carbon cycle. Objective 1:Refine and test a process model for predicting forest carbon dynamics from individual-tree and species-specific traits. This model couples the processes of tree growth, carbon allocation, and mortality, rules for forest canopy interactions, and linkages to climate drivers. Objective 2: To accurately reproduce observed forest dynamics, process model parameter values (“traits”) must be informed by empirical data. Thus, we will continue developing a database of literature information on physiological (e.g., photosynthesis), structural (e.g., woody anatomy), and allometric (e.g., height:diameter) traits for 300+ U.S. tree species. Objective 3: Integrate the literature and existing forest inventory databases with the model to obtain realistic parameter estimates and evaluate the importance of species-specific parameter variability to forest carbon dynamics. This and future work will produce a comprehensive framework that will advance our ability to forecast carbon dynamics across a range of spatial and temporal scales.
Species-specific traits controlling forest and woodland dynamics revealed by Bayesian melding of diverse data and process models. Funded by NSF Biological Informatics.
PI = Kiona Ogle (2006-2007) Summary: The work from study lead to the above (active grants) projects funded by the Wyoming NASA Space Grant Consortium and the NSF program in Advances in Biological Informatics.